Big Data and Public Transit: Measuring Vehicle Trips to Help Modeshift
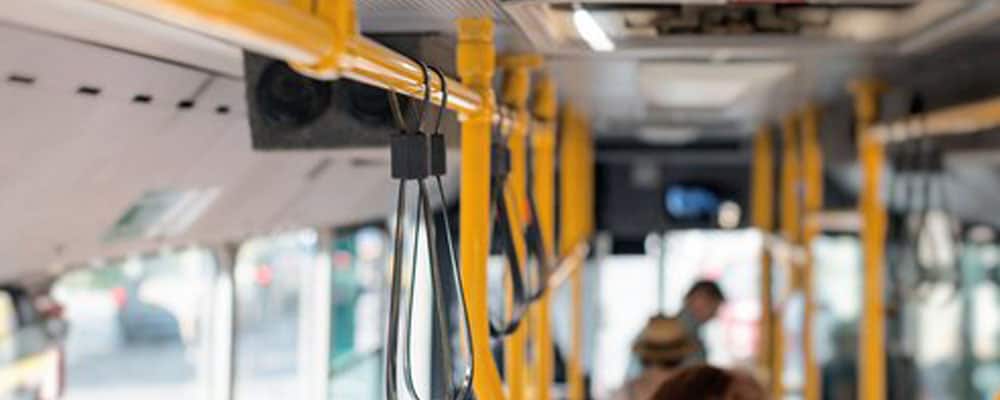
Public transit is a key component of cities’ mobility networks, especially in dense urban centers. Trains and buses help commuters avoid the hassle of traffic jams on congested roadways, not to mention pricey parking. But some cities are attracting commuters and residents so quickly that public transit cannot keep up — just ask anyone who lives in Denver, Colorado.
The population in Denver has grown by ~45% since 1996, and the average commuter there now spends 49 hours per year sitting in traffic, but only 4.4% of commuters use public transit (Source: Denver Post). Similar scenarios are playing out across the US in cities like Austin, Seattle, San Francisco, and more. Even though alternatives to driving are available in many of these growing cities, not enough commuters are using them – and congestion keeps getting worse.
Traditionally, public transit planners improve systems by looking at existing transit users’ behavior. They identify potential users as those who live and work near transit stations. But in this era of rapid urban population growth, we cannot consider these groups alone: What about the people who are driving because transit isn’t currently a viable option? What about the people who could be using the transit to commute, but aren’t? In this blog post, I’ll walk you though a few ways Big Data can help address these questions.
First, let’s define our terms. When we talk about Big Data, we mean the massive volume of location records that are created by mobile devices, connected cars, and connected trucks every day. You can read more about our Big Data sample here. I’m not talking about the type of ridership data that comes from payment and parking systems – most transit planners already have those resources their fingertips.
Big Data is ideal for revealing opportunities for planners to convert vehicle trips to other modes. Here are the key two ways to do this:
- Scan large areas for transit opportunities
- Drill down on target areas to understand transit gaps
Next, I’ll walk you through these two strategies in more detail using a few real-world examples.
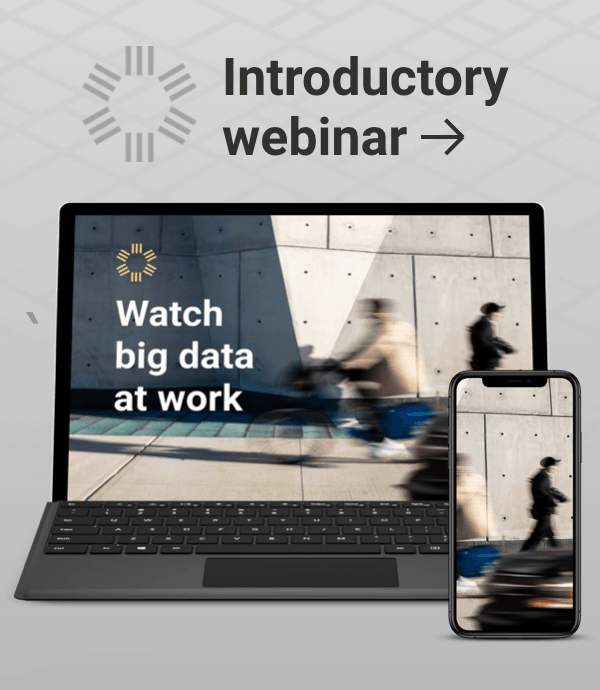
Scanning Large Areas
A key advantage of Big Data is that you can analyze at travel patterns across very large area, such as an entire region or even an entire state. This means you can measure the travel activity of populations that might not respond to your survey – or that you wouldn’t have considered surveying to begin with. Big Data makes it easy to go beyond current transit riders to better understand the travel behavior of drivers – the people that planners are often trying to shift onto transit. To show you how this works, we’ll walk you through a study we created for Denver, CO.
First, we ran large origin-destination (O-D) study of Metro Denver’s ZIP codes in StreetLight InSight, our online platform for analyzing travel behavior with Big Data. (See Figure 1 below.) This type of high-level O-D study can show planners where the greatest number of people start and end their trips. If your goal is to convert as many vehicle trips to transit as possible, it’s best to start with the routes that are most popular.
Figure 1: This heat map visualizes the top origin ZIPs for trips to the Denver Tech Center ZIP, which is outlined in black.
This study quickly revealed that one of the popular destinations of vehicle trips is the Denver Tech Center. It takes about an hour to travel by train from Denver’s downtown train station to the Tech Center. During the morning rush hour, driving takes about the same amount of time due to heavy congestion in the peak period. This offers transit an opportunity to compete for that market.
Our analysis showed that a large share of trips – more than 30% – originate in the ZIPs adjacent to the Tech Center. Investing in bus rapid transit that quickly carries commuters from neighboring ZIPs to the Technology Center should be effective there. Getting those vehicle trips off the road will also help clear the way for drivers that are coming from further a field.
Drilling Down
When people think of “Big Data,” they typically think it’s best for understanding travel patterns at a very high-level; for example, analyzing regional trends and major highways. While that’s absolutely true, these types of studies only scratch the surface of what Big Data can do. Here at StreetLight Data, our navigation-GPS data have 5-meter spatial precision on average, which means we can look at geographies as small as an intersection or off-ramp.
When it comes to public transit, this ability to drill down is really useful. Let’s go back to our study of Denver to illustrate. We know there’s a train in Denver that riders can take right from downtown to the Technology Center in about an hour. Why aren’t drivers taking that train?
To figure this out, we did a TAZ-level analysis of vehicle trips to the Technology Center that take I-25, which follows the route of the train. (See Figure 2 below.)
Figure 2: This shows the origin TAZs of trips to the Denver Technology Center that use I-25 (that’s the purple gate in the lower left of the image) and thus are likely to pass a train station on the way. The blue arrows point to the 5 TAZs with the greatest volume of trips originating there.
When we dug deeper, we also saw that 3 of the 5 TAZs with the highest volumes of vehicle trips to the Tech Center during peak AM hours have either a high school, middle school, or elementary school. It’s very likely that parents are dropping their kids off at school before proceeding on to the Denver Tech Center, and it would be difficult for parents to get their kids to school on the train.
With this type of information, planners can turn to innovative tactics to encourage transit adoption – for example, they could talk to local schools about coordinating parents to carpool to work after dropping their kids off. They could also incentivize this type of behavior with more high-occupancy vehicle or “carpool” lanes.
Another issue could be insufficient transit connections from some of the high-volume TAZs to the train. By investing in more bus, bike, and pedestrian options that make it easy to get to the train, planners can make it more convenient and efficient for drivers to switch to transit.
Putting It All Together
As we’ve demonstrated with our analysis of Denver, Big Data can reveal the key places for transit planners to prioritize expanding their systems. It can also help show why some drivers that could use transit alternatives don’t. But that’s just the beginning.
Big Data can also be helpful for identifying where first-mile and last-mile solutions are most needed – and which types of solutions are the best. Want to learn how we can help with those types of challenges? Let us know in the comments below!