Need Ride-Hailing Services Intel? Big Data Has That.
Need Ride-Hailing Services Intel? Big Data Has That.
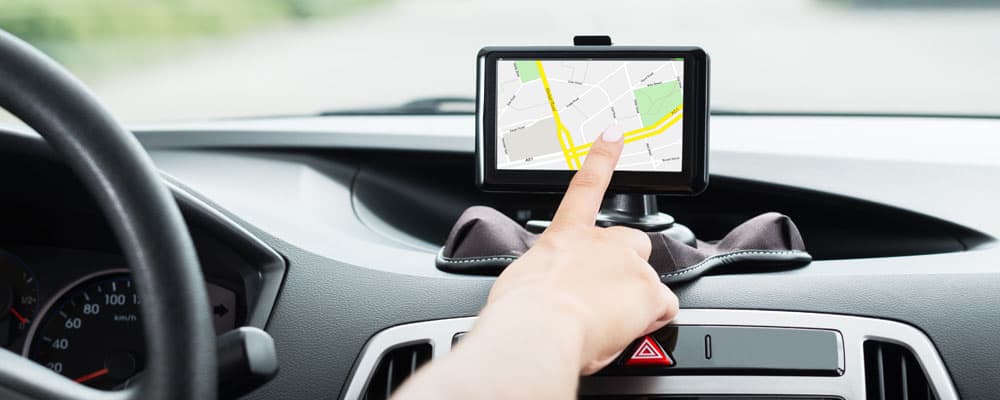
The ride-hailing revolution launched by Uber and Lyft has expanded to embrace shuttles, autonomous vehicles, and micro-mobility options like scooters and bike share.
As ride-hailing services expand, car ownership is decreasing, putting pressure on original equipment manufacturers (OEM) in the automotive industry. Many transportation and mobility companies are working with us to explore business options in this emerging industry.
Planning a ride-hailing business requires researching the target market to understand the best mobility options with strong business potential, and accurately forecasting revenue. But how can a company do that in a business where no data about core patterns exists outside of a few dominant companies who don’t data share? Big Data to the rescue.
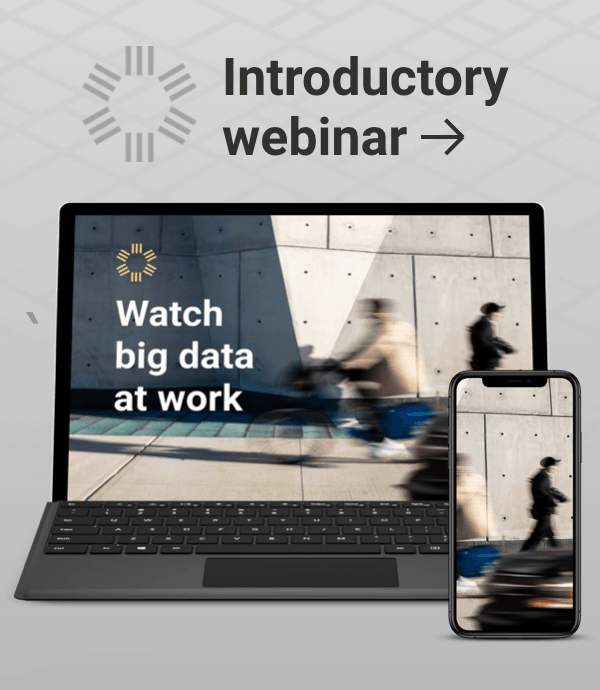
The Data Behind Ride-Hailing Services
What companies entering this new field want is granularity, with accurate data to flow into a model for predictive analysis. Then they can simulate the best business outcomes and opportunities.
For example, key topics for planning a ride-hailing service include:
- Macro considerations: If we start a ride-hailing service, should we launch in Orlando or Dallas?
- Micro considerations: Which neighborhood and routes should we deploy in first? Where should we build assets (such as charging parking garages for a fleet of autonomous cars)? Where should we make costly investments such as HD mapping, and work to get city permissions for test routes? What size vehicle should we manufacture – how many seats, how much cargo space, etc?
StreetLight Data has been working with mobility companies to help answer these questions. With our metrics on ride-hailing service movements, our customers are able to build complex demand models, and help identify opportunities for related businesses.
Available Data and How to Get It
StreetLight is the only reliable, geospatially accurate source of ride-hailing data and non-ride hailing transportation all in one place. In fact, even Uber and Lyft don’t have good data on non-ride-hailing transportation, such as people traveling in their own cars, riding their own bikes, or with other transportation operators. Complete data on all modes is necessary to understand current demand and latent (unsatisfied) demand for new transportation offerings.
How can StreetLight estimate this closely guarded ride-hailing service behavior? Essentially, we can analyze how the movement patterns of devices belonging to ride-hailing drivers differ from other drivers. The patterns are clear: Ride-hailing service vehicles travel more miles per day, via irregular routes. Armed with detailed data, we then use machine learning and probabilistic modeling to apply these “gig driving” patterns/characteristics to our existing database of millions and millions of Location Based Data (LBS) from mobile devices.
As a result, we can offer an unprecedented array of analytics about ride-hailing behavior, including origins and destinations, the volume of ride-hail service drivers on different route segments, the neighborhoods where ride hailing is more or less concentrated, and more.
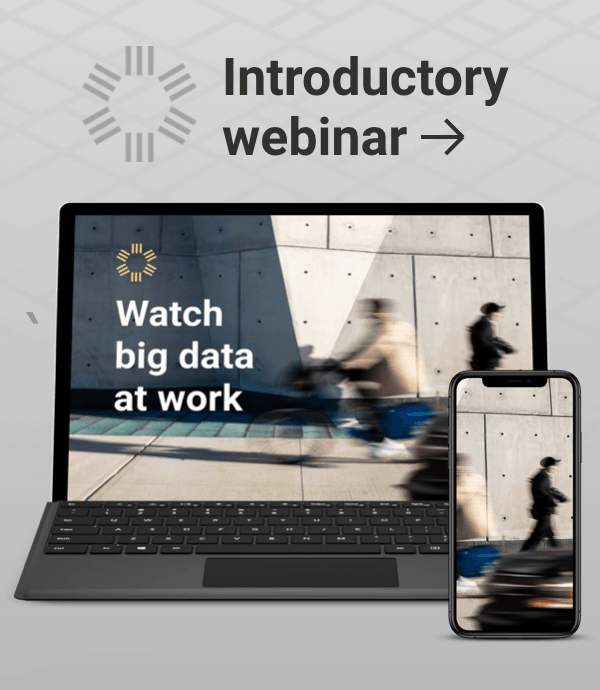
How Companies Use Ride-Hailing Data
Early adopters of our data have run analyses to identify various ride-hailing service patterns such as: Where are the busiest ride hailing routes? How does that change according to time of day and type of day (i.e. weekdays vs weekends)? Where are common clusters of origins and destinations? Are there common “spine” routes that ride-hailing service drivers take, even if the origins and destinations are different?
We not only have data on ride-hailing driving, we can also illuminate, with high spatial granularity/specificity, the difference between ride-hailing services and normal driving. That allows us to spot trends. One obvious example is with nightlife hot spots: we know that ride hailing as a percentage of total driving increases dramatically on Friday and Saturday late nights.
Our customers feed data into their forecast models that use their own proprietary info and assumptions (such as their customers’ willingness to pay, vehicle costs, deployment costs, etc). By layering their business intelligence with our metrics about demand (current and latent) for their future services, they can reveal what investments would be the most lucrative.
For example, consider testing the hypothesis of whether a six-seater shared-ride AV shuttle is more cost-effective to operate than a two-seater, non-shared shuttle. StreetLight’s metrics can show where in a city six people are most likely to share a trip to and from, along with which route(s) to take where people already have proven a willingness to use ride-hailing services.
Looking for granular data about fast-evolving travel behaviors? Don’t expect to get that anytime soon from ride-hailing service companies. Fortunately, Big Data has the answers, and can help companies make informed strategic decisions.