Why Big Data Analysis Matters for Traffic Congestion Studies
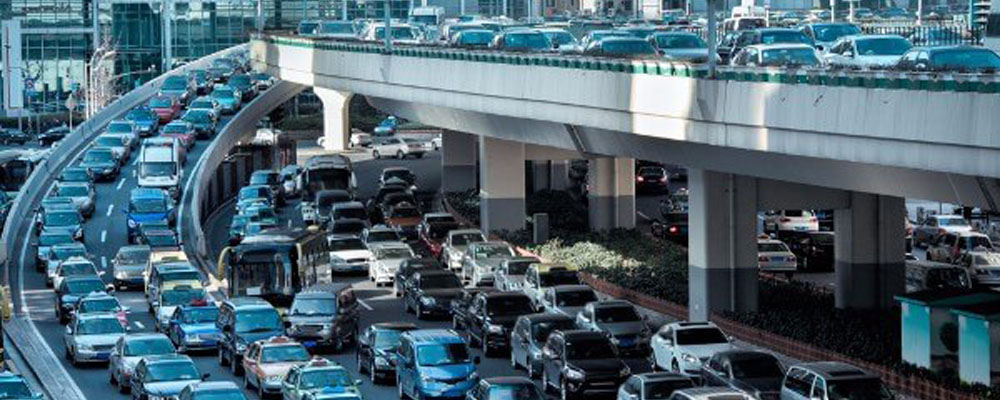
Traffic congestion negatively affects the economy, roads and our quality of life. Some people tend to blame pass-through trips, commercial attractions or employers, but expectations don’t always line up with hard data about what really causes congestion.
In California’s Napa County, for example, it is common to blame traffic on wine-tasting tourists. However, when planners used Big Data to map out travel behavior in traffic congestion studies, they found out that commuters actually contributed as much to congestion as tourists. High housing costs in Napa are a major part of the problem.
It’s not easy to figure out why congestion happens, especially in downtown districts. Learn how to improve the accuracy of your traffic congestion studies by using analytics derived from Big Data.
The Challenge of Traffic Congestion
Traffic congestion occurs whenever the demand for your transportation system outpaces its capacity. Whether you’re vacationing in wine country or getting through the daily commute, the negative effects of traffic congestion are myriad, but not always obvious.
Congestion can make people late for appointments, adds wear and tear to vehicles, and can cause many drivers to be irritable and aggressive. Beyond those typical issues with congestion, it also has serious impacts on the economy (in the form productivity loss), the road surface integrity and the environment.
Trying to reduce congestion without understanding why a specific traffic jam is occurring is a doomed endeavor. The key questions to address include:
- Where is this traffic going to and coming from?
- Who is in this traffic jam?
- Why are they on the road right now?
- What are their other alternatives?
Once gathered, this data measuring traffic congestion can be used by transportation planners and public agencies to identify problems, propose countermeasures, assess improvements and develop policies. But gathering and analyzing this data effectively is no small feat.
Traditional Vs. Big Data Approaches to Traffic Congestion Studies
Let’s look at one typical cause of congestion – roadway repairs. To best manage congestion caused by repairs, planners should make detour routes that help travelers avoid the construction. But just rerouting everyone to the nearest parallel roads will cause its own jam! Looking at the full origin-destination patterns of current users can suggest broader detours, and help direct where to send educational materials about the construction.
In addition, if much traffic in the affected area shares a destination, such as a group of workplaces, the planners can collaborate with those workplaces to offer work-from-home or carpool solutions for the duration of construction. Lastly, if the planners can analyze who is being most affected by the traffic, they know if one socio-economic group is being disproportionately affected, and plan support accordingly.
Unfortunately, it is difficult to gather this data at a truly comprehensive level from traditional data sources. For example, if you are unsure of the destination of congestion, how do you know whom to survey? Surveying everyone in the region would be infeasible and expensive.
In contrast, Big Data provides an affordable, easy resource for understanding travel behavior.
For transportation and urban planning, the phrase “Big Data” can mean a variety of things. In this article, we’re talking about a specific type of Big Data: the location records created by hundreds of millions of mobile devices, such as smartphones, connected cars, connected trucks, and wearables. With the right algorithmic processing techniques, the anonymous, archival and messy data from these devices can be turned into useful information that unlocks great value for transportation engineers and planners.
Analytics based on Big Data allows you to quickly evaluate:
- The top origins and destinations of drivers that use congested routes,
- Levels of congestion and viability of alternative routes,
- The times of day, types of day, and times of year that have the most and least congestion, and
- The purpose of the trips that generate congestion.
Big Data Sources for Congestion Studies
Let’s look in more detail at how Big Data is used in to identify origin-destination (O-D) matrices that contribute to congestion. Using Massive Mobile Data for these matrices can help deliver the fine resolution needed for accurate traffic congestion studies.
When transportation experts have access to data about trips from millions of mobile devices, they can create O-D matrices that represent a far greater percentage of the population, and a longer time span, than could be captured through surveys or license plate studies. O-D data is created by:
- Identifying the pings that occur when mobile devices begin moving (the origins);
- Following the series of pings that occur on the devices’ journeys (the route); and
- Identifying the final pings when devices come to rest (the destinations).
This trip data can be combined with contextual data sets, such as parcel boundaries and demographic information. This means your traditional O-D matrices can be analyzed in terms of trip purpose, opening new insights into the causes of traffic congestion — and the appropriate countermeasures to manage it.
With the use of Big Data and new technology, transportation experts have a huge opportunity to use empirical data for every project – regardless of scale. In the past, transit data collection occurred through travel surveys, but “response rates for travel surveys are…typically low—for example, the response rate for the ARC Regional Travel Survey (2011) varied from 5.9% to 34% across counties,” according to a study done at the Georgia Institute of Technology. It’s important to note the 5.9% to 34% response rate cited in the study reflects the number of households that were selected for the survey and chose to participate. Out of a total regional population of over 1.8M in 2011, the survey collected data from just 10,278 households – that’s just .55%.
This study goes on to say that, “The short-term immediacy of big data makes it useful and compelling for real-time management applications.” The speed and accessibility of Big Data can help planners make more informed decisions, and actually see how their constituents are moving in a more realistic way. Also, conclusions derived from Big Data can be more persuasive than survey data to stakeholders, helping planners get their message across.
Beyond Traffic Congestion Studies
As we’ve discussed, Big Data is an essential tool for understanding the ways behavior changes during specific conditions, and building accurate traffic congestion studies. But there are several other applications that are transforming transportation.
Here are seven other ways to harness the power of Big Data in your planning and projects:
- Transportation Demand Management
- Internal/External Studies
- Travel Demand Modeling
- Project Performance Evaluations
- Performance Measures
- Detour Planning
- Public Transit Design and Expnsion
These use cases are just the beginning. As Big Data evolves and the transportation industry gains experience with this new data source, the use cases could expand greatly.